Case study background and problem formulations
minimizing Cvar_comp_abs (CVaR Absolute Norm)
subject to
Ax ≤b (multiple linear constraints representing convex polyhedron set)
Box constraints (lower bounds on variables)
——————————————————————–——————
Cvar_comp_abs = CVaR component absolute
Box constraints = constraints on individual decision variables
——————————————————————–——————
———————————————
Alpha =0.8 , Solver Precision = 4
———————————————
Data and solution in Run-File Environment
Problem Datasets | # of Variables | # of Rows | Objective Value | Solving Time, PC 2.83GHz (sec) | |||
---|---|---|---|---|---|---|---|
Dataset1 | Problem statement | Data | Solution | 100 | 100,000 | 0.10589 | 2.95 |
Dataset2 | Problem statement | Data | Solution | 100 | 500,000 | 0.10834 | 15.32 |
Dataset3 | Problem statement | Data | Solution | 100 | 1,000,000 | 0.11259 | 29.79 |
Dataset4 | Problem statement | Data | Solution | 100 | 2,000,000 | 0.11259 | 148.05 |
Dataset5 | Problem statement | Data | Solution | 100 | 5,000,000 | 0.11370 | 464.88 |
Dataset6 | Problem statement | Data | Solution | 100 | 9,000,000 | 0.11370 | 829.81 |
Dataset7 | Problem statement | Data | Solution | 500 | 20,000 | 0.01783 | 6.53 |
Dataset8 | Problem statement | Data | Solution | 500 | 50,000 | 0.01828 | 13.13 |
Dataset9 | Problem statement | Data | Solution | 500 | 100,000 | 0.01828 | 32.75 |
Dataset10 | Problem statement | Data | Solution | 500 | 500,000 | 0.01856 | 113.04 |
Dataset11 | Problem statement | Data | Solution | 500 | 1,000,000 | 0.01856 | 303.28 |
Dataset12 | Problem statement | Data | Solution | 500 | 1,500,000 | 0.01856 | 475.0 |
Dataset13 | Problem statement | Data | Solution | 500 | 1,800,000 | 0.01856 | 489.68 |
Dataset14 | Problem statement | Data | Solution | 1000 | 20,000 | 0.00866 | 18.62 |
Dataset15 | Problem statement | Data | Solution | 1000 | 50,000 | 0.00867 | 34.40 |
Dataset16 | Problem statement | Data | Solution | 1000 | 100,000 | 0.00877 | 87.67 |
Dataset17 | Problem statement | Data | Solution | 1000 | 200,000 | 0.00878 | 113.81 |
Dataset18 | Problem statement | Data | Solution | 1000 | 500,000 | 0.00878 | 431.14 |
Dataset19 | Problem statement | Data | Solution | 1000 | 900,000 | 0.00878 | 443.57 |
NOTE: Problem statements can be simplified using MultiConstraint.
Data and solution in MATLAB Environment
Problem Datasets | # of Variables | # of Rows | Objective Value | Solving Time, PC 3.50GHz (sec) | |||
---|---|---|---|---|---|---|---|
Dataset1 | Matlab code | Data | Solution | 100 | 100,000 | 0.10589 | 2.85 |
———————————————
Alpha =0.9, Solver Precision = 7
———————————————
Data and solution in Run-File Environment
Problem Datasets | # of Variables | # of Rows | Objective Value | Solving Time, PC 2.83GHz (sec) | |||
---|---|---|---|---|---|---|---|
Dataset1 | Problem statement | Data | Solution | 100 | 100,000 | 0.10589 | 4.89 |
Dataset2 | Problem statement | Data | Solution | 100 | 500,000 | 0.10833 | 25.23 |
Dataset3 | Problem statement | Data | Solution | 100 | 1,000,000 | 0.11259 | 41.27 |
Dataset4 | Problem statement | Data | Solution | 100 | 2,000,000 | 0.11259 | 160.24 |
Dataset5 | Problem statement | Data | Solution | 100 | 5,000,000 | 0.11370 | 442.18 |
Dataset6 | Problem statement | Data | Solution | 100 | 9,000,000 | 0.11370 | 847.96 |
NOTE: Problem statements can be simplified using MultiConstraint.
Data and solution in MATLAB Environment
Problem Datasets | # of Variables | # of Rows | Objective Value | Solving Time, PC 3.50GHz (sec) | |||
---|---|---|---|---|---|---|---|
Dataset1 | Matlab code | Data | Solution | 100 | 100,000 | 0.10589 | 4.79 |
Dataset2 | Matlab code | Data | Solution | 100 | 500,000 | 0.10833 | 24.73 |
Dataset3 | Matlab code | Data | Solution | 100 | 1,000,000 | 0.11259 | 40.14 |
——————————————————
Alpha =0.955279, Solver Precision = 7
——————————————————
Data and solution in Run-File Environment
Problem Datasets | # of Variables | # of Rows | Objective Value | Solving Time, PC 2.83GHz (sec) | |||
---|---|---|---|---|---|---|---|
Dataset1 | Problem statement | Data | Solution | 500 | 20,000 | 0.01783 | 21.83 |
Dataset2 | Problem statement | Data | Solution | 500 | 50,000 | 0.01828 | 40.46 |
Dataset3 | Problem statement | Data | Solution | 500 | 100,000 | 0.01828 | 94.30 |
Dataset4 | Problem statement | Data | Solution | 500 | 500,000 | 0.01855 | 717.9 |
Dataset5 | Problem statement | Data | Solution | 500 | 1,000,000 | 0.01855 | 1295.71 |
Dataset6 | Problem statement | Data | Solution | 500 | 1,500,000 | 0.01855 | 1739.97 |
Dataset7 | Problem statement | Data | Solution | 500 | 1,800,000 | 0.01855 | 1643.09 |
NOTE: Problem statements can be simplified using MultiConstraint.
——————————————————
Alpha =0.968377, Solver Precision = 7
——————————————————
Download Problem Data
Problem Datasets | # of Variables | # of Rows | Objective Value | Solving Time, PC 2.83GHz (sec) | |||
---|---|---|---|---|---|---|---|
Dataset1 | Problem statement | Data | Solution | 1000 | 20,000 | 0.00866 | 92.55 |
Dataset2 | Problem statement | Data | Solution | 1000 | 50,000 | 0.00867 | 273.03 |
Dataset3 | Problem statement | Data | Solution | 1000 | 100,000 | 0.00877 | 408.29 |
Dataset4 | Problem statement | Data | Solution | 1000 | 200,000 | 0.00877 | 1363.49 |
Dataset5 | Problem statement | Data | Solution | 1000 | 500,000 | 0.00877 | 2617.07 |
Dataset6 | Problem statement | Data | Solution | 1000 | 900,000 | 0.00877 | 4654.56 |
NOTE: Problem statements can be simplified using MultiConstraint.
minimizing [(1-lambda)*polynom_abs+ lambda*max_comp_abs]
subject to
Ax ≤b (multiple linear constraints representing convex polyhedron set)
Box constraints (lower bounds on variables)
——————————————————————–——————
polynom_abs = polynomial absolute function
max_comp_abs=maximum component absolute function
Box constraints = constraints on individual decision variables
——————————————————————–——————
——————————————————
Lambda =0.90909, Solver Precision = 7
——————————————————
Download Problem Data
Problem Datasets | # of Variables | # of Rows | Objective Value | Solving Time, PC 2.83GHz (sec) | |||
---|---|---|---|---|---|---|---|
Dataset1 | Problem statement | Data | Solution | 100 | 100,000 | 1.01459 | 6.62 |
Dataset2 | Problem statement | Data | Solution | 100 | 500,000 | 1.05439 | 27.53 |
Dataset3 | Problem statement | Data | Solution | 100 | 1,000,000 | 1.06657 | 47.81 |
Dataset4 | Problem statement | Data | Solution | 100 | 2,000,000 | 1.07531 | 168.45 |
Dataset5 | Problem statement | Data | Solution | 100 | 5,000,000 | 1.09822 | 468.64 |
Dataset6 | Problem statement | Data | Solution | 100 | 9,000,000 | 1.10039 | 851.35 |
NOTE: Problem statements can be simplified using MultiConstraint.
——————————————————
Lambda =0.95744, Solver Precision = 7
——————————————————
Download Problem Data
Problem Datasets | # of Variables | # of Rows | Objective Value | Solving Time, PC 2.83GHz (sec) | |||
---|---|---|---|---|---|---|---|
Dataset1 | Problem statement | Data | Solution | 500 | 20,000 | 0.38609 | 35.07 |
Dataset2 | Problem statement | Data | Solution | 500 | 50,000 | 0.38966 | 70.52 |
Dataset3 | Problem statement | Data | Solution | 500 | 100,000 | 0.39170 | 128.08 |
Dataset4 | Problem statement | Data | Solution | 500 | 500,000 | 0.39654 | 744.98 |
Dataset5 | Problem statement | Data | Solution | 500 | 1,000,000 | 0.39868 | 1571.72 |
Dataset6 | Problem statement | Data | Solution | 500 | 1,500,000 | 0.39956 | 2574.43 |
Dataset7 | Problem statement | Data | Solution | 500 | 1,800,000 | 0.40005 | 2909.3 |
NOTE: Problem statements can be simplified using MultiConstraint.
——————————————————
Lambda =0.96923, Solver Precision = 7
——————————————————
Download Problem Data
Problem Datasets | # of Variables | # of Rows | Objective Value | Solving Time, PC 2.83GHz (sec) | |||
---|---|---|---|---|---|---|---|
Dataset1 | Problem statement | Data | Solution | 1000 | 20,000 | 0.26782 | 161.54 |
Dataset2 | Problem statement | Data | Solution | 1000 | 50,000 | 0.26938 | 318.55 |
Dataset3 | Problem statement | Data | Solution | 1000 | 100,000 | 0.27064 | 475.09 |
Dataset4 | Problem statement | Data | Solution | 1000 | 200,000 | 0.27165 | 1209.68 |
Dataset5 | Problem statement | Data | Solution | 1000 | 500,000 | 0.27194 | 3165.31 |
Dataset6 | Problem statement | Data | Solution | 1000 | 900,000 | 0.27194 | 5612.34 |
NOTE: Problem statements can be simplified using MultiConstraint.
minimizing quadratic (square of L_2 norm)
subject to
Ax ≤b (multiple linear constraints representing convex polyhedron set)
Box constraints (lower bounds on variables)
——————————————————————–——————
quadratic = quadratic function
Box constraints = constraints on individual decision variables
——————————————————————–——————
——————————
Solver Precision = 7
——————————
Download Problem Data
Problem Datasets | # of Variables | # of Rows | Objective Value | Solving Time, PC 2.83GHz (sec) | |||
---|---|---|---|---|---|---|---|
Dataset1 | Problem statement | Data | Solution | 100 | 100,000 | 1.03483 | 2.47 |
Dataset2 | Problem statement | Data | Solution | 100 | 500,000 | 1.11188 | 15.83 |
Dataset3 | Problem statement | Data | Solution | 100 | 1,000,000 | 1.14674 | 30.70 |
Dataset4 | Problem statement | Data | Solution | 100 | 2,000,000 | 1.15551 | 134.57 |
Dataset5 | Problem statement | Data | Solution | 100 | 5,000,000 | 1.20912 | 324.14 |
Dataset6 | Problem statement | Data | Solution | 100 | 9,000,000 | 1.21178 | 914.29 |
Dataset7 | Problem statement | Data | Solution | 500 | 20,000 | 0.15307 | 4.30 |
Dataset8 | Problem statement | Data | Solution | 500 | 50,000 | 0.15612 | 21.83 |
Dataset9 | Problem statement | Data | Solution | 500 | 100,000 | 0.15752 | 25.26 |
Dataset10 | Problem statement | Data | Solution | 500 | 500,000 | 0.16135 | 115.8 |
Dataset11 | Problem statement | Data | Solution | 500 | 1,000,000 | 0.16346 | 291.92 |
Dataset12 | Problem statement | Data | Solution | 500 | 1,500,000 | 0.16393 | 335.87 |
Dataset13 | Problem statement | Data | Solution | 500 | 1,800,000 | 0.16425 | 388.55 |
Dataset14 | Problem statement | Data | Solution | 1000 | 20,000 | 0.05064 | 0.63 |
Dataset15 | Problem statement | Data | Solution | 1000 | 50,000 | 0.05064 | 0.94 |
Dataset16 | Problem statement | Data | Solution | 1000 | 100,000 | 0.05064 | 1.50 |
Dataset17 | Problem statement | Data | Solution | 1000 | 200,000 | 0.05064 | 2.57 |
Dataset18 | Problem statement | Data | Solution | 1000 | 500,000 | 0.05064 | 5.87 |
Dataset19 | Problem statement | Data | Solution | 1000 | 900,000 | 0.05064 | 162.24 |
NOTE: Problem statements can be simplified using MultiConstraint.
This case study considers the projection problems with various norms on a polyhedron set
given by a system of linear inequalities. In particular, we consider the Scaled CVaR
Absolute Norm introduced in Pavlikov and Uryasev (2013). The Scaled CVaR Absolute Norm
in


so-called confidence level, controlling conservativeness of the norm. CVaR term and the
optimization approach for CVaR was introduced in Rockafellar and Uryasev (2000). For the
most conservative case,

value of components of the vector. The least conservative norm, with

values of all components. In the intermediate case, for



the norm of a vector is defined as the average of

components. If


convex combination of two values of the norm with nearest to

of

Computational experiments for
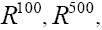

sets

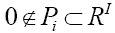
projection of 0 on


average of


with confidence level

solves the projection problems with CVaR Absolute Norm for dimensions

and confidence levels,
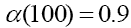


confidence levels, in this case, were selected with the formula

to the best approximation of

(2013). Problem 2 solves projection problems with weighted average of


dimensions





make the best approximation of the



norms, i.e.,



Problem 3 solves projection problems with square of


• Pavlikov K, and S. Uryasev (2013): CVaR Absolute Norm and Applications in Optimization.
Research Report # 2013-1.
• Rockafellar, R.T. and S. Uryasev (2013): The Fundamental Risk Quadrangle in Risk
Management, Optimization, and Statistical Estimation. Surveys in Operations Research and
Management Science, 18 (to appear).
• Rockafellar, R. T. and Uryasev, S. (2000), “Optimization of conditional value-at-risk”,
Journal of Risk , Vol. 2, pp. 21–41.
• Gotoh, J. and S. Uryasev (2013): Approximation of Euclidean norm by LP-representable
norms and applications. Draft paper.